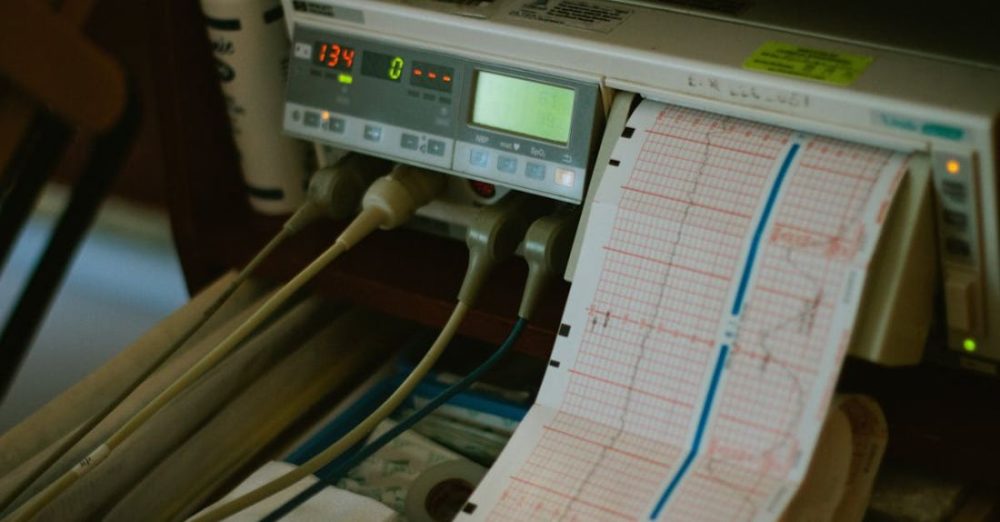
In recent years, machine learning has emerged as a powerful tool in the field of computational biology, revolutionizing the way researchers analyze and interpret complex biological data. By leveraging algorithms and statistical models to identify patterns and make predictions from large datasets, machine learning is driving significant advancements in understanding biological systems and processes. Let’s delve into how machine learning is transforming computational biology.
**Enhanced Data Analysis**
One of the key areas where machine learning is making a significant impact in computational biology is in data analysis. Traditional methods for analyzing biological data often struggle to handle the sheer volume and complexity of information generated by modern research techniques such as high-throughput sequencing. Machine learning algorithms, on the other hand, excel at processing large datasets and extracting meaningful patterns and insights. By training models on labeled data, researchers can classify genes, proteins, and other biological entities with high accuracy, leading to a deeper understanding of biological systems.
**Predictive Modeling**
Machine learning enables researchers to build predictive models that can forecast outcomes and trends in biological processes. By training algorithms on historical data, scientists can develop models that predict, for example, the impact of genetic mutations on protein function, the response of a tumor to a particular drug, or the likelihood of developing a specific disease based on genetic markers. These predictive models not only enhance our understanding of complex biological phenomena but also have the potential to guide personalized medicine and drug discovery efforts.
**Drug Discovery and Development**
The application of machine learning in computational biology has the potential to significantly accelerate the drug discovery and development process. By analyzing vast amounts of chemical and biological data, machine learning algorithms can identify potential drug candidates, predict their efficacy, and optimize their properties for specific targets. This approach, known as virtual screening, allows researchers to quickly screen large libraries of compounds and prioritize those with the highest likelihood of success, saving time and resources in the drug development pipeline.
**Biological Image Analysis**
Another area where machine learning is driving innovation in computational biology is in the analysis of biological images. With the increasing use of imaging technologies such as microscopy and medical imaging, the volume of image data being generated is growing exponentially. Machine learning algorithms trained on labeled image data can automatically segment cells, detect patterns, and classify structures within images with high accuracy. This not only speeds up the analysis process but also enables new discoveries in fields such as cell biology and pathology.
**Challenges and Opportunities**
While machine learning holds great promise for transforming computational biology, it also presents challenges that need to be addressed. One of the key challenges is the need for high-quality, labeled training data to train accurate models. Additionally, interpreting the results of machine learning algorithms and ensuring their reliability in biological applications require domain expertise and careful validation.
**Innovations on the Horizon**
Looking ahead, the integration of machine learning with other cutting-edge technologies such as single-cell sequencing, spatial transcriptomics, and CRISPR-based gene editing holds immense potential for driving further advancements in computational biology. By combining these technologies, researchers can gain deeper insights into cellular processes, disease mechanisms, and drug responses, ultimately leading to more personalized and effective treatments.
**The Future of Computational Biology**
In conclusion, machine learning is revolutionizing computational biology by enabling researchers to analyze large datasets, build predictive models, accelerate drug discovery, and advance biological image analysis. While challenges remain, the opportunities for innovation and discovery in this field are vast. As machine learning continues to evolve and mature, its integration with other technologies promises to unlock new frontiers in our understanding of the complexities of biological systems. The future of computational biology is bright, driven by the transformative power of machine learning.